I wrote my first post on this blog at the start of 2008 when I was a 22 year old university student. Prime time for a quarter life crisis, which I think was a term people were already joking about back then. Almost exactly 14 years later on the last day of 2021, I am a 36 year old, have a faculty position at a university, and tenure.
It’s hard to put myself back in the mindset of that 22 year old, but I do remember spending a lot of time wondering what I was going to do with my life. I’d gone to college as an econ major. Switched to biology because I liked my hourly job working in a lab. Skipped a lot of class but mostly* managed to pull As and Bs by picking courses that focused on midterms and finals, not classwork/homework. As graduation approached, the econ majors were all interviewing for jobs at big NYC investment banks. I remember a guy I knew rushing back into class after taking a call: “They offered me a higher salary than my dad makes!” But what do you do with an undergrad degree in biology? I wasn’t sure. One thing I did know a person could do with an undergrad degree in biology was get a GRADUATE degree in biology. I was majoring in biology because I liked working in a lab, so grad school seemed like a reasonable next step. I applied. I got in. I went.
In retrospect it was very good I didn’t stay an econ major. The fall of 2008 was not a good time to be starting in investment banking. What with the world financial system melting down and all.
In any case: I got in my old car and drove 2,800 miles (almost all of it on I-80) from New York to California** and grad school. I was bad at some parts of it. Good at others. I joined a lab that let me play to my strengths. Published my first paper. Then another. And another. Got a lollipop (as is tradition) and a diploma. What do you do with a PhD in biology? In principle a number of things, but the main path you hear about is to get a postdoc. That’s what about 70% of people who get a PhD in biology do next. I got one of those. And after a postdoc? Well there is lots of competition, but some people are able to get tenure track faculty positions. About 15% of biology PhDs. Although that overstates how hard it is, since there are plenty of people who get a PhD but would run away screaming from the idea of being a professor (and hence don’t apply for those jobs). Anyway. I got one of those jobs. What do you do with a tenure track faculty position? You put your head down and you work hard and you try to get tenure. When it comes to getting tenure, working hard is necessary-but-not-sufficient. I worked hard, but I was also really fortunate to have great students and creative collaborators and good luck. In any case. I got tenure.
What do you do with a tenured faculty position?
I’m serious, what do you do? For the first time since switching my major from economics to biology in 2005 (when I was all of 19 years old), I don’t have an obvious default Next Step. At least beyond: Do more of the same stuff you did to get tenure.
In the field people call this either a “post-tenure slump” or “post-tenure depression.” Apparently lots of folks go through it although fewer people talk about it in person. In seems to share many characteristics with a midlife crisis (e.g. “what am I doing with my life?”).
I found out about the decision about tenure in 2019. Really if I was going to have a post-tenure slump or depression it should have happened in 2020. As it turned out, my attention, like almost all of ours, was focused on other things in 2020. And the first part of 2021. But this summer and fall it has really started to sink in.
In principle, I could spend the next twenty to forty years of my life doing the exact same stuff I did over the past five. And if I did that it would probably been enough to keep this job until I’m old enough to retire. And if my job ends up going away it is more likely to be a result of the overall health of the university I work for, a factor I have extremely limited capacity to influence.*** It’s a good situation to be in and it’s also terrifying.
For the first time in my adult life, I am not facing a major hurdle in the coming years where, if I don’t buckle down and work hard enough, I’ll be forced off my current trajectory and have to reevaluate my life (not getting into grad school, failing my qualifying exam and leaving with a masters, not getting a job offer, not getting tenure). If I’m going to reevaluate my life, I have to decide myself to do so. It won’t be forced upon me. My goal for 2022 is to do a little of that reevaluation.
Ideally I’d like come up with one or more answer to how to spend the next 20-40 years that feels a bit more appealing/fulfilling/meaningful than continuing to focus the vast majority of my energy, attention, and time on a job where I work on the same questions with the same methods.**** I’m not sure what answer does appeal, but I know that answer doesn’t.
Anyway, that’s my 2022 goal: To come up with some goals to have. In the meantime I have to go work on filling out my annual report.
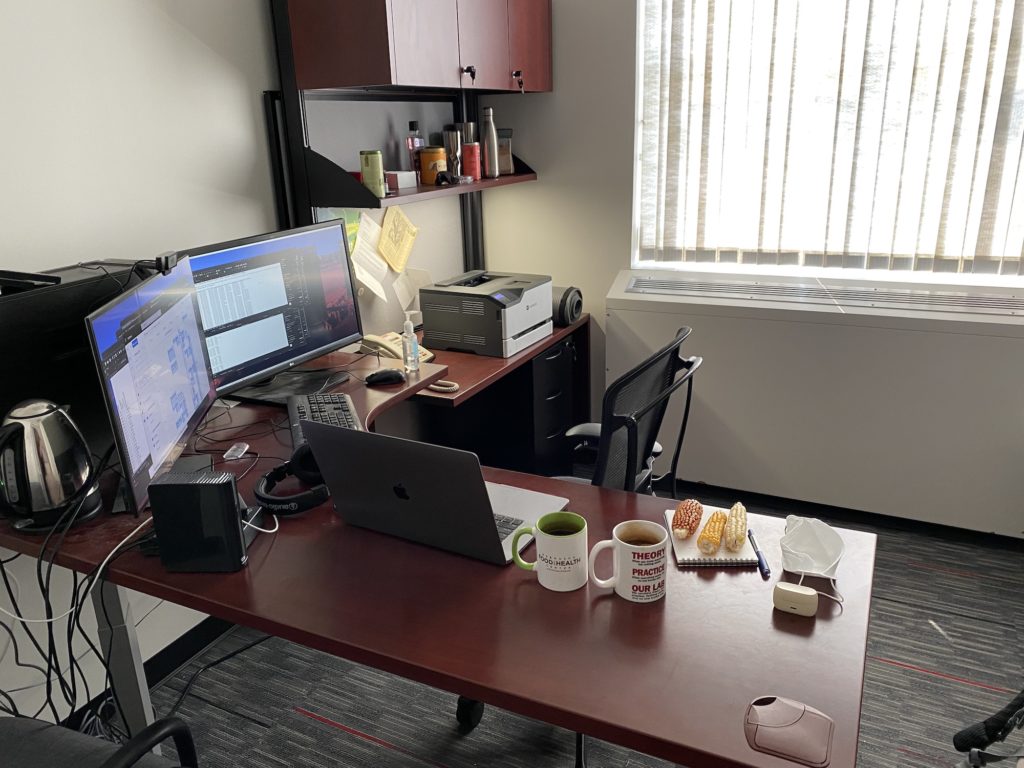
*General Chemistry and Organic Chemistry being notable exceptions. Frankly I’m lucky I graduated at all.
**In fairness there was a multi-month stop for the summer at my folks house in the middle of that, so it wasn’t 2,800 miles straight through.
***Declines in student enrollment, both domestic and international, are real. I don’t know what the international situation will look like in ten years but looking at the demographics domestic enrollment pretty much has to decline going forward because there are are fewer 14-year-olds than 15-year-olds in this country, fewer 13-year-olds than 14-year-olds, and so on.
****I’m also trying to make my peace with the fact that, no matter how hard I work or how much I accomplish in a given, so long as I have this particular job my department is still going to tell me every year that I still didn’t do enough stuff. It’s surprisingly hard for me to accept. I think I’m doing a good job. I think I am perceived outside the university as doing a good job. I think even inside the university when it boils down to it, there are people who believe I am doing a good job. Yet, even so, being told each year that I’m not doing enough work has worked its way under my skin to an extent that embarrasses me and to an extent that is neither healthy nor productive.